Welcome to the Decarb Guidebook
Approach
Benchmarking
Building Codes & Design Standards
State & Local Regulations
Technologies: Load Reduction
Technologies: Dehumidification
Technologies: If you must have a gas-fired boiler
Technologies: Central Plant
Technologies: Domestic Hot Water
Technologies: Steam
Technologies: Load Shifting and Energy Storage
Technologies: Emerging Technologies
Motivation Program
Pilot Projects
How to Pay for Decarbonization
Community Discussions
Workshops
Study 1: Historical Cooling Loads Analysis
Background
A team was tasked with analysis of historical loads at multiple hospital sites. Their goal was to document cooling loads. A full presentation of the project process and results can be found on YouTube.
The project was also peer-reviewed and presented at ASHRAE in the conference paper Sanborn et al (2021) “Determining Cooling Loads in Health Care Clinical Spaces Using Historical Data”.
Setup of the project
The project was intended to be the be first of its kind. The project team transparently explained their data collection and data analysis methods. It is hoped other teams can replicate and improve upon these efforts.
The project occurred between August and December 2021. The goals were:
1. Assess historical peak sensible cooling load for several room functions.
2. Assess the diversity of simultaneous sensible cooling loads for several room functions.
Facilities included in the project are shown in Table 1. The facilities included were limited by the project timeline and budget. These facilities were those where the team could assemble both cloud-based diagnostic data and zone and room design data.
Facility Name | Facility Type | IECC Climate Zone | Climate Zone, CEC | ASHRAE Design 0.4% Outdoor Air Dry-Bulb (°F/°C) | Years of Trended Data | Actual 0.4% Outdoor Air Dry-Bulb (°F/°C) |
San Leandro Medical Center | Hospital | 3C – Marine | 3 | 83.9/28.8 | 2020 | 97.9/36.6 |
Modesto Medical Center | Hospital | 3B – Hot/Dry | 12 | 101.7/38.7 | 2020 | 109.8/43.2 |
Riverside Cirby Medical Office | Medical Offices | 3B – Hot Dry | 11 | 101.8/38.8 | 2020 | 105.8/41.0 |
South Bay Medical Center | Hospital | 3B – Hot/Dry | 6 | 91.8/33.2 | 2020 | 92.9/33.8 |
Table 1: Facilities Included in the Study
Cloud-Based Diagnostic Data
Trended point data came from a cloud-based continuous commissioning and fault detection diagnostic platform. Site building automation systems (BAS) are connected to the platform. All BAS points are pulled into the platform at 5-minute intervals. These points are never deleted. For each zone, the team downloaded a comma-separated data file with columns for (a) Timestamp, (b) Supply Air Volume Rate, (c) Room Air Temperature, and (d) Supply Air Temperature. The supply air volume rate and supply air temperature are from zone air terminal unit sensors. The supply air temperature is sensed after the zone reheat coil. The room air temperature is from a wall-mounted zone thermostat. Each file was approximately 100,000 rows of data. The team downloaded 827 zone files, containing more than 84 million records. For each site, the team downloaded a comma-separated data file with columns for (a) Timestamp, and (b) Outdoor Air Temperature.
Room and Zone Attribute Data
To be assessed, each zone needed to be mapped to its design details. The team compiled databases including (a) zone identification, (b) function of the room with the thermostat, (c) area of the room with the thermostat, and (d) total area served by the zone. Where possible, the team gathered additional data for: (a) peak design airflows (b) design cooling loads (c) interior or perimeter (d) floor number, and (e) direction of the façade.
The team dropped some zones from the sample if the zone served disparate spaces. For the results in Table 2 and Table 3, the zones included primarily served spaces with the same function as the room with the thermostat.
Data Collection and Aggregation
The team had to clean up the data for comparison. Data had different timestamp formats. Each BAS system had different naming for the points. Design data came from different sources. From the timestamps, the team calculated (a) month, (b) hour of the day, and (c) day of the week. The team merged each zone’s diagnostic data with the average hourly outside air temperature. All calculations were performed in IP units.
Zone sensible load was calculated for each time interval with this equation: Qs (Btu/h) =1.08*CFM*ΔT, where “CFM” represents zone supply air volume rate and “ΔT” represents the delta between zone supply air temperature and room air temperature. Net hourly loads were aggregated from the sensible load calculations in that hour. The team limited the assessment to cooling loads. After calculating net hourly load, the team dropped all heating hours (net hourly loads greater than zero). Loads were divided by the area of the zone (in ft2) and expressed as Btu/hr-ft2.
The Zone Sensible Cooling Load results are presented using percentiles (See Table 2 in the Results section). The team identified the 75%, 50%, 25%, 2%, and 0.4% exceedance levels. The team used percentiles in lieu of reporting mean and standard deviation, since the load data is not normally distributed. Using standard deviations as a measure of outliers is only applicable if the data fits a normal distribution, an example of which is shown in Figure 2. Additionally, there is precedent for the use of percentiles in cooling load calculations. ASHRAE Handbook of Fundamentals lists design weather conditions as percentiles to ensure they represent the same probability of occurrence regardless of seasonal distribution in temperature.
The team performed quantile-quantile (Q-Q) analysis to assess normality and found it lacking. Q-Q analysis compares the quantiles of a given dataset against those of a theoretical normally distributed dataset. Figure 1 shows an example histogram for the Zone Type “Patient Room”. The team discovered that load data is non-normal. Figure 2 shows a quantile-quantile plot, to test for normality in a sample set of room load data. The load data consistently fails. The major implication of this is that standard deviations should not be used. Rather, percentiles are the more appropriate expression of load distributions.
Evaluation of Diversity
The team evaluated diversity by finding the maximum simultaneously occurring sensible cooling load in multiple zones of the same Zone Type (See Table 3 of the Results.) This is not air handling unit supply air or cooling coil diversity; however, zone type diversity can be used to inform duct main and shaft sizing.
For most zone types, there were no hours where all zones in the sample, Ntotal, were cooling during the same hour. Figure 3 shows the variation in the number of zones that are simultaneously cooling for the Patient Room zones. The largest number of zones cooling in the same hour, Nmax-cooling, is shown, with that hour. Interestingly, the hour where Nmax-cooling occurs is also not the same hour where Qblock-max, the largest simultaneous hourly cooling load summed across all zones of the same type, occurs. Where: Qblock-max= MAX (ΣQN) and QN, or Qblock, represents the coincident sensible cooling load of a zone type at a given hour. Qblock-max is shown in the table, with its hour. Zone Type Diversity, Dzone-type, is expressed as a function of the peak single-zone load from the sample, Qzone-0.4%peak. In Equation 1 below the term, Qzone-0.4%peak * Ntotal , represents the sum of the peak zone cooling load for a given zone type.
(1)
It is worth noting that while Qblock-max and Qzone-0.4%peak are shown in Btu/h-ft2 in Table 3, the calculation above was performed with Btu/h units.
Other Analysis Details, Checks and Pre-Results
Outdoor air temperature (lack of strong relationship). The results (in Table 2 and Table 3) include all cooling hours from May to October, for the hours of 8 AM to 6 PM. At the project start, the team had goals to separately analyze “neutral hours” for perimeter spaces. “Neutral hours” would be those with outdoor air temperatures between 67°F and 76°F (19°C-24°C). The team assumed it would be simple to identify a strong relationship between peak load and weather. However, these analyses were not immediately conclusive. There was a strong, relevant, and reportable relationship between cooling load and time-of-day. However, analysis thus far has not revealed a strong and reportable relationship between cooling load and outdoor air temperature.
2020 versus 2019 (check for differences). Given that the time window for our analysis was 2020, coinciding with the COVID-19 pandemic, we performed a comparative analysis with 2019 data for a subset of zones. We selected ten patient zones in San Leandro and compared hourly loads between 2019 and 2020. We used the same monthly and hourly constraints. We found a difference between the mean hourly loads for 2019 and 2020, 4.4 Btu/h-ft2 to 4.7 Btu/h-ft2, respectively. While this is statistically significant, the difference is not a large one relative to cooling load planning. Additionally, the differences in loads were unrelated to visiting hours (before 6 am and after 7 pm), which had been hypothesized.
Diversity Across Sites. To help ensure that diversity calculations, shown in Table 3, were not unique to San Leandro Medical Center, the same calculations were performed for patient rooms at South Bay Medical Center as well as Modesto Medical Center. Patient rooms were selected for this comparison, as this zone type had the greatest available sample size across all sites. As anticipated, patient rooms in South Bay and Modesto tended to match those in San Leandro. While actual values varied due to different conditions across sites, trends followed a similar downwards pattern. As the number of simultaneously cooling zones increased, diversity decreased.
Façade direction: In figure 5, the zones at the San Leandro hospital are divided by façade direction. There are two sets of bars: red for all zones, and blue for patient rooms. For all zones, façade direction has the expected, fairly clear influence in the overall zone cooling loads. The ENE and WSW façades have higher peak loads than the ESE, NNW, SSE, or SSW façades. However, for the patient rooms, this effect is radically diminished. Peak loads in the patient rooms are much lower than the rest of the building, and variance is only 1 – 2 BTU/hr-sf. This indicates that the type of zone is much more important than the façade direction.
Interior vs exterior zones: Interior zones seem to have, as a rule, higher cooling loads than perimeter zones. And, the outside air temperature has very little impact on variance in loads
Results
The figure below shows the results for Zone Sensible Cooling Load from multiple sites. Results were calculated in BTU/hr-ft2. Converted values are shown in W/m2
Violin plots
For easier visualization, the above data is also plotted in the violin plots. In this form, it becomes visually very clear that the peak load is very much not representative of the most common conditions.
Block loads for zone types
The team assessed block loads for each type of zone. Importantly, this is not a block load diversity that an air handler would see. Rather, this is an assessment of simultaneous load occurring in multiple instances.
Diversity values here were somewhat shocking to the team. Many design engineers assume 100% diversity, where all loads are included at all times in all rooms. The data shows that assumption is likely wildly conservative, even if only two or three of the same zone type exists in the facility.
Study 1: Conclusions and Recommendations
At the close of this work, the team recommended the following:
This data in Figure 6 should be used to check the quality of load calculations and energy models. For reasonably similar climates, calculated loads which are greater or less than those identified here should be scrutinized. Likewise, if an energy model shows a profile of cooling loads that varies significantly from the percentiles, the inputs should be scrutinized. The diversity factors shown in Figure 9 should be used to check the sizing of systems which serve more than one of the various load types. The data indicates a great deal of diversity occurs. Many engineers assume 100% diversity, which will result in oversized equipment.
Study 2: Hospital Energy Modeling Inputs
Background
A second team looked specifically at hospital spaces. In a three week effort, in January 2022, the objective was to look at energy modeling inputs critically, considering the sources of data for those inputs. The full study is here on YouTube.
The focus of the effort was patient rooms, ICU patient rooms, operating rooms, imaging rooms, and corridors and nurses stations. Corridors and nurses stations were assumed to be similar, and were clustered together into one category.
Data Availability from Recent Hospitals
At the start of the effort, the team intended to gather as much data as possible from recently constructed hospitals with submetering. There were two recently constructed hospitals designed by the same team with lighting sub-metering (two sites), plug loads (one site), HVCAC data (one site), and elevators (one site).
However, in many cases, the submetering was inoperable, or non-responsive, or incorrectly mapped. This, in itself, is a major lesson learned. Investments in sub-metering need to be fully commissioned to have value. Data needs to flow where it is needed.
Previously Published Studies for Comparison
As most of the data from the recently constructed sites was not in usable form, the team relied heavily on previously published studies. Those studies included the work of Team 1, as well as previous studies published by ASHRAE and Targeting 100. Targeting 100’s study of energy end uses in a hospital (Hatten, “Legacy Salmon Creek”) is the only published study of actual loads in a running hospital. The loads measured were used to inform the input schedules for the modeling of the Targeting 100 studies. Targeting 100’s input schedules are the nearest thing the industry has to a use-based of input schedules. ASHRAE 90.1 also has input schedules, which are an industry standard. However, their origin and basis is not well documented. There are no available reports indicating they have been validated by actual data. It is likely these schedules represent an “engineering judgment” about what plug, lighting, and occupancy schedules ought to be.
Process
The team’s process is illustrated below.
Patient Rooms
The team wanted to match the overall occurrence of loads in patient rooms over the course of a year. Reviewing the work of Team 1 (above), they determined the best target to aim at was the percentile data from 67 patient rooms. The overall goal, then, became to find a set of inputs that yielded similar 25%, 50%, and 75% loads which were reported by Team 1.
The team studied a variety of input schedules. The first check was a simple visual check, shown below for 15 different scenarios tested (an additional 14 were also tested). The team plotted the resultant histogram of modeled cooling loads, and visually compared it to the historical loads (the “San Leandro” histogram in the top left corner). A recurrent problem is that an energy model’s distribution is more normal (tending towards an average), whereas the historical data is non normal, weighting heavily to the low end.
A second method of comparison was the Kolmogorov-Smirnov 2 sample test, a statical method to determine if two non-normal samples are likely from the same distribution. Shown below, if any p value is below 0.05, the conclusion is that the two samples are not from the same distribution. Under this test, all of the modeled distributions failed. (I.e. None of them were similar enough to the actual data to pass a statistical evaluation.) However, the deviation (indicated by the D value), was lower for some that for others.
In the end, the team chose to select the input distribution based on the closest match of the 25%, 50%, and 75% percentiles. Interestingly, the ASHRAE 90.1 schedules perform reasonably well at the 50% and 75% percentiles, less so at the 25% percentile. The team’s PR8 and PR 9 schedules performed closest across all four percentile markers.
The final, selected input schedule is shown below, with the resultant percentiles, as compared to the actual data.
This first study, of patient rooms, yielded one of the most surprising finds of the work. The shape of the input schedule needed to match the historical data, with it’s two steep mountains, is far from intuitive. The same schedule is also recommended for ICU patient rooms, since both the source data and the resultant profiles are similar.
Operating Rooms
The two previous studies of operating rooms produced different data. The KP Internal Study data on operating rooms was a smaller sample size, and produced a much lower overall BTU/hr-sf range. The team selected the internal KP study as the target distribution.
The visual check of operating room schedules is shown below.
The statistical tests, and percentile tests are shown below
The recommended values, and the resultant distribution of loads are shown below
Imaging Rooms
For imaging rooms, in addition to the Team 1 data, there was an ASHRAE conference paper (citation needed) detailing the load profile for two weeks in summer. This data showed a fairly constant, low level load, with infrequent peaks, comparted to the overall Team 1 data. Both were used for comparison.
The visual check is shown below. Since the source data for the targeted load profile was limited, the statistical and percentile checks were not applicable.
The recommended input values are shown below, with the resultant load occurrences. The selected schedule was a modification of an input schedule from Targeting 100.
PACU
With less data available, and a smaller sample size, the team
The sample size for PACU was not huge
Corridors and Nurses Stations
Working under the assumption that corridors and nurses stations are blended as a common space, the team calibrated an input schedule to the Team 1 percentile data.
Fan Power
The data from the San Diego Medical Center, which is a constant-volume chilled beam hospital was collected, and is shown in Figure 1.
Figure 2 shows the relationships between fan power, fan flow, and outside air temperature. The ranges of fan power found in the data are consistent with expectations. Many of the systems (e.g. AHU-2 and AHU-7) are constant volume systems, which makes the data validation fairly straightforward.
The team’s conclusion on fan power was that the modeling appeared to be relatively accurate. The model for the new building (KPSJ) showed levels about 10% higher, but, the team did not feel this needed to be corrected.
Study 2: Conclusions & Recommendations
The input schedules from available sources, such as ASHRAE 90.1 and Targeting 100 have some value, in the absence of other data. However, when quality data is available from similar use cases, it should be compared to the modeling results.
Study 3: Clinic Energy Modeling Inputs
Background
A third team convened in late 2022 to form recommendations on the modeling inputs for clinic buildings. The full study is here on YouTube.
The effort had an historical forensic opportunity: to compare actual load data to a basis of design on a recently constructed building.
The Hesperia MOB is a 55,000 ft2 templatized medical office building, with a high level of project and historical analytical information.
1. The design team of the building documented their energy model assumptions, energy model outputs, and a cooling load profile (see figure X) into the project’s basis of design.
2. For the same building, the retroactive team had access to historical cooling load data, using the methods of analysis discussed in the previous sections (see figure).
Checking for a representative building
The team looked at several recently opened clinic buildings, to determine if the Hesperia MOB could be treated as a representative.
The figure below shows the Hesperia MOB historical cooling loads, versus six other recent projects. All are shown in the same format as figure 1. It was determined that the Hesperia building was a conservative representative. The peak historical loads were in line with other projects, or higher. The load profile was higher than others projects.
One load profile or many
At the starting point, the team noted that the median loads for the most common clinic space types are quite similar.
Based on this observation, the team decided to treat the majority of clinical spaces under one load profile.
Calibrating the Energy Model to the Actual Data
An energy model was run for the Hesperia building, and directly compared. The iterative process is illustrated in Figure 1.
The Basis of Design assumptions were well documented.
At the first glance, the energy model produced higher overall loads, and higher load profiles than the historical data. See figure 1.
Diversity factors were systematically lowered for the energy model, until a reasonable correspondence was achieved at 50% diversity factor. See figure 2
The team hesitated to recommend a 50% diversity factor, since 50% diversity factor will most likely terrify most design engineers. Rather, the team recommended sizing equipment based on a 60% to 70% diversity factor, which would correspond to the actual load (50% diversity) plus a reasonable (15% - 20%) factor of safety.
Looking at Modeling Schedules
Design engineers, in the process of creating energy models and load calculations, must input the occupancy, lighting, and equipment load profile schedules. This is a key part of the process, which the team sought to validate.
In addition to the historical load data, the team noted that the building had already been profiled in Google maps, which offered an interesting insight as to the occupancy. While not showing numbers of occupants, or occupants per unit area, the data indicated two peaks at ~10am and 2-3pm.
The team looked at the original schedules, the load based schedules, and the insight from google maps together in figure 3
The resultant yearly derived schedule is the recommended schedule from this team. Similar to the hospital team in part 2, the recommendation is to use the same schedule for all assumed load factors (occupancy, lighting, and equipment) since there is currently no basis for differentiating.
Validation of the Load Histogram
As a check, the team looked at the resultant energy model load histogram versus the historical load histogram. See figure 3
Overall Cooling Load Profiles for Outpatient Spaces
Table 1 above was updated based on data from three outpatient buildings. For Offices and exam rooms, this sample size is much larger than table 1.
The load percentiles are shown graphically, for the Hesperia MOB data, in this figure.
Study 4: Internal KP Study on Operating Room Loads
Background
Kaiser Permanente did a study of operating room loads, as part of an evaluation of decoupled systems (See sidebar). with the primary goal of understanding the maximum simultaneously occurring load in a set of rooms. (The study also looked at temperatures and setbacks in the room data. The full study is available on YouTube.
Zone | Building | Equipment | Notes |
F001 | Antioch Sand Creek Hospital | CAV H-2-6-29 OR-3 H-7 | 2,000 cfm zone; OR |
F002 | Antioch Sand Creek Hospital | CAV-H-2-6-23 OR-1 | 2000 cfm zone; OR |
F003 | Antioch Sand Creek Hospital | CAV-H-2-6-30 OR-2 | 2000 cfm zone; OR |
F004 | Antioch Sand Creek Hospital | OR-4 Rm H2458 | 2000 cfm zone; OR |
F005 | Antioch Sand Creek Hospital | OR-5 Rm H2459 | 2000 cfm zone; OR |
F006 | Antioch Sand Creek Hospital | OR-6 Rm H2462 | 2,400 cfm zone; OR |
F007 | Antioch Sand Creek MOB 2 | CAV S-2-5-15 GENERAL OR 2560 | 2000 cfm zone |
F008 | Antioch Sand Creek MOB 2 | CAV S-2-5-18 GENERAL OR 2570 | 2000 cfm zone |
F009 | Antioch Sand Creek MOB 2 | CAV S-2-5-21 GENERAL OR 2630 | 2300 cfm zone |
F010 | Antioch Sand Creek MOB 2 | CAV S-2-5-24 GENERAL OR 2640 | 2100 cfm zone |
F011 | Antioch Sand Creek MOB 2 | CAV S-2-5-26 GENERAL OR 2650 | 2200 cfm zone |
F012 | Carson ASC | CAV-1-S-60, OR-3 | 1680 cfm zone |
F013 | Carson ASC | CAV-1-S-62, OR-4 | 1680 cfm zone |
F014 | Carson ASC | CAV-1-S-64, OR-2 | 1680 cfm zone |
F015 | Carson ASC | CAV-1-S-66, OR-5 | 1680 cfm zone |
F016 | Carson ASC | CAV-1-S-68, OR-1 | 1680 cfm zone |
F017 | Carson ASC | CAV-1-S-70, OR-6 | 1680 cfm zone |
Table 1. Operating Rooms Collected for Load Analytics
Data was gathered from seventeen operating rooms, in three buildings. For each zone, one year of 5-minute interval was collected (1/1/2020 to 1/1/2021). The points collected were: zone airflow (in CFM); room temperature (in F), and supply air temperature (in F). In total, there were 105,000 observations per room.
Loads were calculated for each hour of historical data, using the simple expression in Figure 1:
The average load, by hour of the day is shown in Figure 1. The overall, or annual average, load is shown in Figure 2 below. For most of the rooms, load rises at 6 or 7 in the morning, and ramps down from 3 in the afternoon on. There's are some rooms that are different than that. Several of these rooms stay between 0 and 9,000 BTU/hr of load. The highest hourly average loads are 23,000 BTU/hr. On an annual basis, one room has an average as high as 18,000 BTU/hr.
Maximum and minimum loads for the rooms are shown in Figure 3. The maximum hourly load, in any operating room, was 37,400 BTU/hr. The minimum load is typically negative, indicating a heating condition.
Maximum Coincident Load and Diversity for Number of Rooms
The purposes of the study was to look at the maximum simultaneously occurring load in an array of rooms, as a guideline for sizing cooling units, as well as shafts and air handlers in a decoupled system for multiple rooms.
The single hour maximum is about 275,000 BTU/hr in the 17 rooms, which amounts to 16,000 BTU/hr per room. 90% of the coincident loads are below 125,000 BTU/hr, which is less than 7,300 BTU/hr per room of coincident load.
The data was also analyzed based on the number of rooms. For each hour, the rooms with the highest loads were summed. This resultant diversity factor against a single-room maximum load is shown in Figure 5.
Overall Distribution of Loads
The entire data set was then sorted into a histogram of load, in BTU/hr-sf, which is shown in Figure 5. The maximum of the data set was 64.5 BTU/hr-sf.
Internal KP Study: Conclusion and Recommendation:
The maximum simultaneous cooling load occurring in a set of 17 operating rooms, for 90% of observations, was below 7,300 BTU/hr. The maximum (100%) simultaneously occurring load in a set of 17 operating rooms was 16,000 BTU/hr per room. Diversity of peak loads can be planned, based on Figure 5.
Sidebar: Clean Room Concept of Operating Rooms
Most operating rooms in US hospitals are served by full-sized air handlers. The traditional approach to HVAC for operating rooms is to use one or more air handlers to serve the rooms. Those air handlers move the full airflow required by each room, which is typically 20 ACH or higher. Since operating rooms always have less cooling load that full cooling at 20 ACH, reheat coils are placed per room, to add heat.
Energy intensity in operating rooms is high, based primarily on the large air quantities and the constant need for reheat. Under simple modeling, operating rooms have an energy intensity of approximately 1,000 kBTU/sf-yr, 65% to 70% of which is the reheat energy (See Figure). Any strategy that could reduce reheat energy could significantly reduce operating room intensity.
An alternative approach to operating room HVAC is to use local recirculating fans, with terminal filtration, coupled with a DOAS system providing cooling, outdoor air, and pressurization. The DOAS unit can be sized for a fraction of the total air capacity. Reheat load can be significantly reduced, and, in many hours, entirely eliminated.
Overall Recommendations and Future Trends
The work detailed here suggests several paths forward:
Design engineers who are interested in accuracy of their load calculations and energy models should be fluent in data analytics. As BAS data moves to cloud-based systems, historical data will become more and more available. Fault-detection and diagnostic systems offer a significant opportunity for accessing large data sets of historical data.
Sample sizes should grow with time.
Load incident data is non-normal. As such, analysis of hourly loads and energies should never use expressions of mean and standard deviation. This analysis recommends that percentiles be used to avoid this problem. (For HVAC engineers, percentiles and exceedance hours should be familiar, since this is similar to the way weather data is expressed.)
Actual lighting loads and plug loads should be further studied. To date, apart from the Targeting 100 study in 2008, a whole hospital’s worth of lighting data or plug load data has not been evaluated. Plug load measurement schemes, even where applied, have not yet yielded useful data for validation of the modeling and load calculation assumptions. The lighting profiles in Targeting 100 are most likely obsolete, since they pre-date the large scale adoption of LED technology.
Occupancies in hospitals have not been studied in a systematic way. Load calculations and energy models can contain assumptions near 100 sq.ft. per person for a hospital, while the sum of staff and 20 visitors at any one time is closer to 300 sq.ft. per person. Until some assumptions are published, design engineers should always validate total occupancy of a model against the parking number. In the absence of other guidance, that peak occupancy should assume an 80% full parking lot at one person per car, in addition to the bed count of the hospital itself.
A future analysis might be undertaken to understand if, in fact, the overnight equipment loads in ICU patient rooms is significantly higher across a sample than non-ICU patient rooms. At the moment, there is not analysis to suggest the distinction has any significance.
Comments
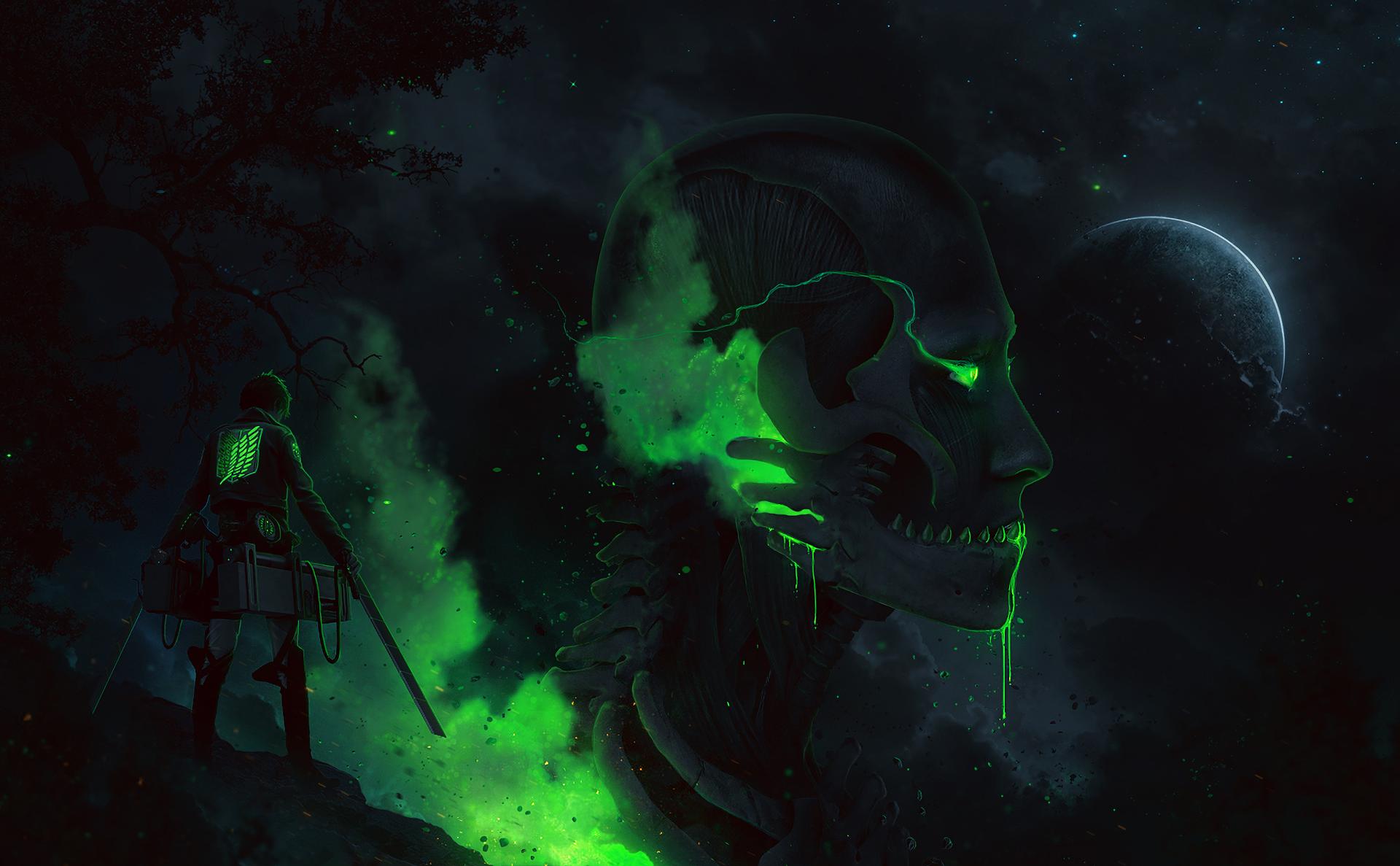
John Doe
8 seconds ago
Lorem ipsum dolor sit amet consectetur adipisicing elit. Quisquam, voluptatum. Lorem ipsum dolor sit amet consectetur adipisicing elit. Quisquam, voluptatum.
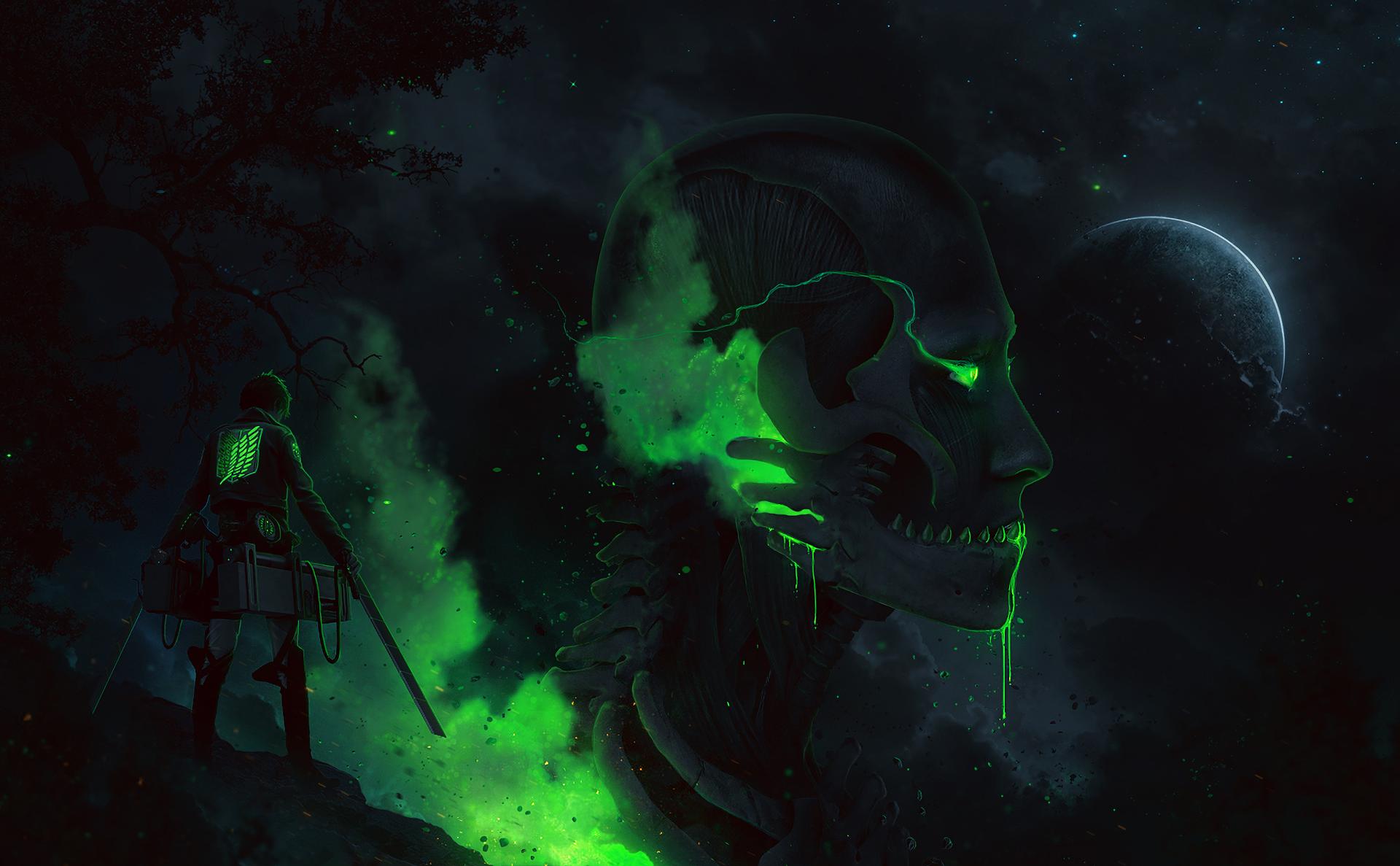
John Doe
18 seconds ago
Lorem ipsum dolor sit amet consectetur adipisicing elit. Quisquam, voluptatum. Lorem ipsum dolor sit amet consectetur adipisicing elit. Quisquam, voluptatum.